Oh noes!
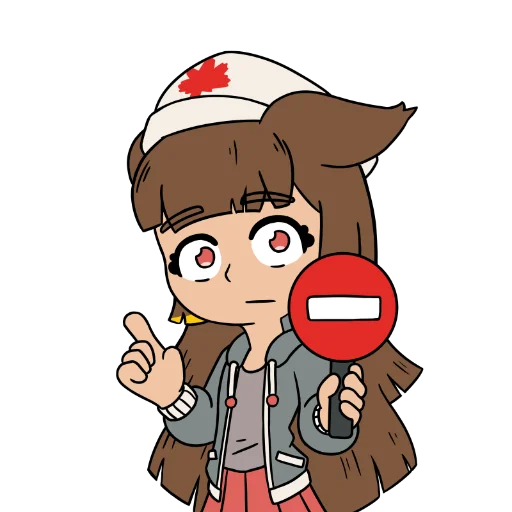
Access Denied: error code 26301432c34cf028.
Go home or if you believe you should not be blocked, please contact the webmaster at webmaster@cringe-studios.com
Access Denied: error code 26301432c34cf028.
Go home or if you believe you should not be blocked, please contact the webmaster at webmaster@cringe-studios.com